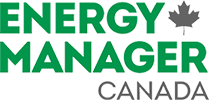
Features
AI and Improving Your Building’s Performance
Deciphering artificial intelligence and understanding how new analytics systems will lead to better, smarter, buildings.
May 13, 2020 By Ricardo Moromisato
These days it’s hard to avoid hearing about or reading news articles or online postings related to Artificial Intelligence, or AI. Whether it is smart appliances and home automation systems with intelligent digital assistants, autonomous vehicles, advanced medical diagnostics, virtual reality, or even human-like automatons—there seems to be no stopping AI. And when it comes to buildings, new automation systems used to control comfort, security and energy efficiency are moving towards intelligently controlled solutions.
History of AI
AI is not a new concept. In fact, it was coined over 60 years ago by John McCarthy during a conference with fellow scientists at Dartmouth College in New Hampshire. Yet AI, described as the ability for machines to simulate intellectual processes characteristic of humans, is an area of study that has existed long before its inception as an academic discipline. Alan Turing, the British mathematician known for his cryptanalysis of the Enigma during the Second World War, and whose life is featured in the 2014 film, “The Imitation Game”, invented his “a-machine” (automatic machine) in 1936, and is widely considered to be the father of theoretical computer science and AI.
Clarifying Terminology
Even in our world of facility and energy management, AI sits among a long list of buzzwords including data mining, machine learning, predictive analytics, data science, deep learning, and many others which are trending upwards and gaining renewed popularity. However, even when these words are springing up in our everyday conversations and media discussions, for many of us the concepts are often fuzzy at best and incomprehensible at worst.
This article aims to provide some clarity to this ‘word salad’, giving basic definitions and explaining how these concepts relate to each other, and when it comes to smart buildings, suggesting that the most suitable and all-encompassing term is probably predictive analytics. The article will also answer the question: “How can predictive analytics help save energy and improve occupant comfort?”
The diagram below shows how the various disciplines, methodologies, processes and applications relate to each other. Obviously, there are many different points of view on how these concepts are defined, inter-related and applied. Through careful research, this diagram is an attempt at developing a simplified framework to help explain some key ideas.
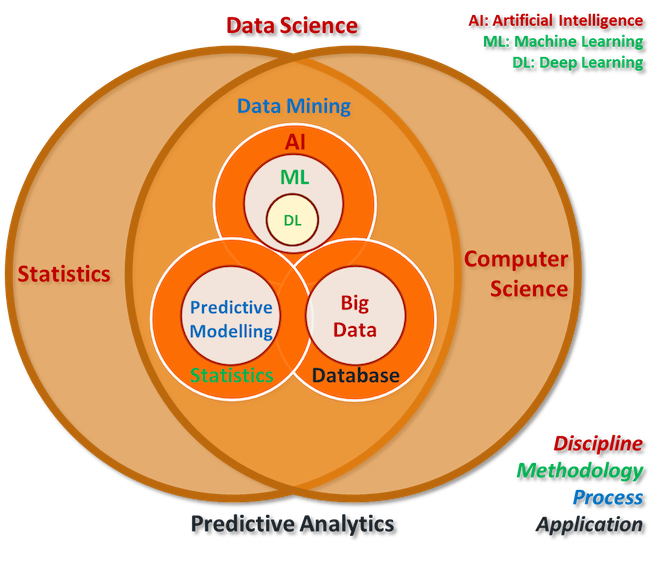
Figure 1: Data Science: disciplines, methodologies, processes and applications – and their relationship to each other. (CopperTree Analytics)
In essence, figure 1 depicts the multidisciplinary field of data science with its two primary disciplines, statistics and computer science, represented by the two largest intersecting circles. Data mining, shown at the intersection of both disciplines, is typically understood as an interdisciplinary process involving AI, statistics and database systems.
Machine Learning (ML) is widely accepted as a methodology and subset of AI (others include natural language processing, machine vision, etc.) hence represented as a circle inside AI, with Deep Learning (DL) being a subset of ML, represented by the smallest circle contained within.
Predictive modelling, shown inside the circle representing statistics methodologies, is defined as a process tightly coupled with statistics to predict outcomes. Database systems commonly refer to the software applications that organize data for analysis, with big data, the related field that handles data sizes exceeding traditional hardware and software, represented by the inner circle. Predictive analytics is generally understood as an application of data science requiring its inter-related disciplines, methodologies and processes to generate the expected outcomes.
Predictive Analytics
In a nutshell, predictive analytics is the analysis of historical information to find patterns useful in making informed predictions about future events. This type of evaluation is achieved using data mining processes and ML techniques with a variety of algorithms including decision tree regression, Bayesian networks, genetic algorithms and Artificial Neural Networks (ANN) – a deep learning subset of ML. It’s indeed the basis for Fault Detection and Diagnostics (FDD) methods based on process history. In the built environment, having a robust Building Automation System (BAS) is a great start for collecting process history, as systems connect and retrieve building data on a variety of open and proprietary protocols, including BACnet as well as vendor-specific protocols.
But… is it actually possible to predict the future by looking back at history? According to an article in The Economist, “Does the past predict the future?”, “…historical data remains the best way to forecast the future… Historical data may be imperfect, but it remains the only unbiased way to measure risk and make assumptions about the future.”
Predictive analytics is definitely playing a huge role in Energy Information Systems (EIS) and Automated Fault Detection and Diagnostics (AFDD). Let’s take a closer look at the practical applications aimed at improving building energy use, occupant comfort and overall facility management. One such ‘ingredient’ is predictive modeling, which we defined earlier as a process tightly coupled with statistics to predict outcomes. This is in fact at the core of predictive analytics.
Predictive Modeling
Figure 2 below depicts the modeling process, whereby the model—a mathematical representation of a given system—is created once data is organized. During a training step, the algorithm learns through observed system behavior and is followed by testing and validation. This iterative cycle continues until an acceptable model is obtained, at which time it is deployed to begin making predictions for eventual comparison with measured data. Following are three predictive modeling examples with a few practical applications relevant to buildings.
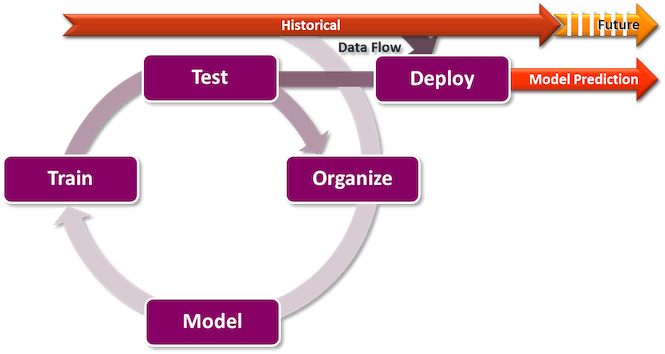
Figure 2: Data flow and predictive modeling iterative process. (CopperTree Analytics)
Energy Baseline Determination
Using historical data to model energy consumption is arguably the most common application of predictive analytics within the building management space. A predictive model of energy use could be developed using independent variables such as weather logs—provided there is a direct correlation with building energy consumption—aided by a linear regression function to determine the line of best fit.
This model would then be fed with historical weather data to estimate energy usage, using it as the baseline to which actual energy consumption is compared against. Another typical application is to use this energy predictor with forecasted atmospheric conditions in order to implement demand limiting strategies to avoid peak demand charges.
Occupancy Prediction
An occupancy model could be developed to predict the number of occupants in a building based on historical data. For example, using historical data from passive infrared (PIR) motion detectors, Wi-Fi device counts, or plug loads, an occupancy profile model could be developed with the help of data mining and machine learning techniques.
Predicted occupancy profiles would then be used to optimize schedules, improve occupant comfort ensuring a smooth transition from unoccupied mode, and calculate the building’s actual outdoor air fraction requirements instead of using the typical 30% minimum outdoor air damper position for ventilation systems—the rule of thumb when a building is fully occupied.
System Characterization
System modeling is another application of predictive analytics, where historical data from temperature sensors and final control elements such as control valves and dampers are used to establish a mathematical model to represent the thermodynamic characteristics of any given system. Modeling approaches include white-box, grey-box, and black-box models, with the former being more physical and principle-based, while the latter being more empirical, thus requiring significant amounts of training data.
Modeled system characteristics would then be used to identify equipment faults, including unresponsive, stuck or leaky control valves and dampers, fans and pumps showing signs of imminent failure, as well as operational inefficiencies leading to optimized control strategies for optimal energy utilization.
Conclusion
Ultimately, the goal of predictive analytics is to improve asset management. And this includes tangible assets such as buildings and equipment, and intangible ones such as human capital, energy, and financial assets. Analytics platforms for improving occupant comfort and building energy efficiency exist today, and developers are constantly enhancing their platforms and services to help building owners along with energy and facility managers achieve those objectives.
Ricardo Moromisato, is a business intelligence analyst, smart buildings, with CopperTree Analytics. Based in Surrey, B.C., CopperTree Analytics provides energy management and automated Fault Detection and Diagnostics with its Kaizen software to optimize the performance, reduce energy consumption and improve occupant comfort in buildings. www.coppertreeanalytics.com/
Print this page